Related articles
Confronting the new healthcare AI revolution
Predicting the future of artificial intelligence in clinical decision support
Medical algorithms may improve or worsen racial and ethnic disparities
Black Americans use different language for depression than white Americans, revealing limitations of AI
AI intervention reduces end-of-life expenses for cancer patients
Large-scale language models (LLMs) are artificial intelligence (AI) systems that understand and generate human language by processing vast amounts of text data. With the launch of ChatGPT in late 2022, an example of an LLM has entered the lives of many Americans.
At the same time, although less flashy, a variety of large-scale language models became commonplace in some healthcare settings, for example by programming chatbots to answer health-related questions.
Technology companies, including Penn, and academic researchers took notice. “Artificial intelligence has not been successfully implemented in the medical field, but with the advent of large-scale language models, the potential to improve care delivery suddenly becomes enormous,” said LDI Senior Fellow Hamsa Hamsa. said Associate Professor Bastani (Operations, Information and Science). Decision at Wharton. A little less than a year ago, Penn opened the Wharton Healthcare Analytics Lab (WHAL) with Bastani and LDI Senior Fellow Marissa King as faculty co-directors. WHAL has one postdoctoral researcher, 10 affiliated faculty members, several graduate students, and an executive director.
The Institute's mission is to help bring advanced data analytics to the medical field. “We want our analytics to have an impact and that impact to be transformative,” said King, a professor of healthcare management at Wharton.
However, there are major obstacles, including disagreements among stakeholders on how to implement the model. “I believe that harnessing the potential of large-scale language models will require everyone from regulators to practitioners to data scientists to come together to overcome the challenges,” King said.
A great starting point, she added, is the October 10 conference “(Re)Writing the Future of Healthcare with Generative AI,” co-hosted by LDI and WHAL. King sees the first-ever symposium as a “neutral forum” where stakeholders can share their concerns and possible solutions.
Although there is great excitement about the potential of LLM in medicine, the lab is taking a cautious and rigorous approach. At the conference, Mr. Bastani will moderate a session called “Drafting the LLM Handbook: Key Questions for the Health System.” She intends to raise informed concerns within WHAL's work and audience on issues such as patient safety and data accuracy, bias and equity challenges, and data infrastructure.
Patient safety and data accuracy
One of the most interesting applications of large-scale language models is using them to populate a patient's electronic health record (EHR) and generate a summary of a clinical visit. “Doctors hate making notes. They got into this profession because they want to look patients in the eye and talk to them about what they're feeling. Doctors don't want to write notes during consultations. I have yet to speak to anyone who is not very excited about the possibility of LLM taking over,” Bastani said.
The problem with LLM is that it can lead to illusions such as false or missing information. For example, a patient with diabetes may be incorrectly tagged as having high blood pressure on a discharge summary from the ER department. This is because the two conditions usually coexist. “These models are extrapolation machines, so they often extrapolate correctly, but they can also extrapolate incorrectly,” Bastani explained. Such mistakes can later lead to therapeutic errors.
Working with Somaliland's Ministry of Health and Development in the Horn of Africa, Bastani and her graduate students have the advantage of working in a health data system that is just beginning to go digital. “We are testing a balanced LLM intervention that reduces physician burden while maintaining high accuracy in clinical notes. A subset of these notes will be randomly audited and reviewed by a practicing clinical expert. We test thousands of things and call it a success if there are only one or two inaccuracies. We want to make sure we're not doing much worse than the rate,” Bastani said.
In the United States, such an intervention may be difficult to pull off. “But if physicians are willing to carefully audit these summaries and treat them as drafts rather than final products, that can be very helpful. So how do we encourage them to act that way? There's a problem: Various technology companies have shown some pretty promising results, but they haven't undergone rigorous academic evaluation,” Bastani said. Additionally, LLMs can be “fine-tuned” by retraining them on new datasets related to the exact tasks they need to perform well.
Bias and fairness challenges
Current thinking suggests that medical data, especially electronic health records (EHRs), is rife with bias. “A big problem with algorithms and equity is that health systems for underserved populations and minorities often don't include high-quality data, resulting in poorer health outcomes for them. “I think the best way to address algorithmic bias is to collect better data if possible,” Bastani said. The “gold standard,” she added, is for health systems to reach out to patients who are unable to make it to the hospital, offering free transportation to and from their appointments, for example. “If we can't do that, there are various tools in machine learning to reduce bias, but they're not as effective as collecting more and better data about people,” Bastani said. .
Biases can come into play even after the model is complete. LDI Senior Fellow Kevin Volpp, director of the Penn State Center for Health Incentives and Behavioral Economics, will work with WHAL on Penn State Medicine's cardiovascular risk reduction initiative to more efficiently direct resources to those at highest predicted risk. We are exploring the use of AI to guide people. “A key question is whether AI will systematically allocate resources to some groups rather than others. “We need to ensure that health inequalities are reduced by improving health outcomes,” he said.
data infrastructure
Large-scale language models provide an unparalleled opportunity to mine data from sources that have not previously been exploited in a systematic way. Mr. King uses his LLM's ability to understand text and emotion to analyze issues related to employee well-being. “One of the things we are trying to do is use data from electronic health records to understand where clinicians, especially nurses, are at high risk for burnout and mental overload. “The records contain very rich data,” she said.
However, “obtaining and building datasets for a project is a daunting task. There is no question that the data challenges associated with AI and healthcare are the biggest impediment to progress,” King said. Masu. All of these and other issues will be the subject of discussion and brainstorming at a joint meeting on October 10th.
Creating a suitable HIPAA-compliant environment that can support training and fine-tuning of LLMs on patient-identifiable notes and voice conversation data will maximize the potential of these technologies to improve healthcare delivery. This is a significant challenge that health systems must overcome before they become obsolete. scale.
author
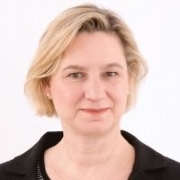
Nancy Steadman
journalist
Learn more about health equity
blog post
health equity
One-third of U.S. gun injuries in 2019 and 2020 were fatal
First-ever national analysis reveals racial and geographic disparities
October 4, 2024
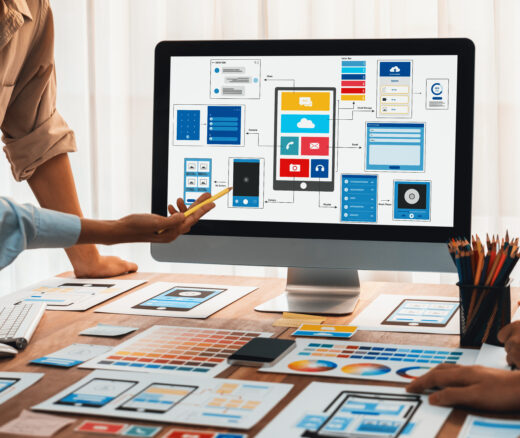
blog post
health equity
Four steps to building apps and other digital health tools ethically
LDI fellows provide concrete ways to avoid disparities and better serve patients
October 1, 2024
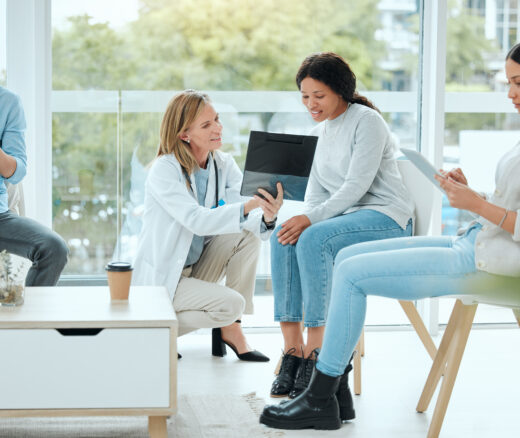
blog post
health equity
Low trust in government can negatively impact clinical trial participation
Evidence from Philadelphia during the pandemic suggests ways to build trust
September 26, 2024
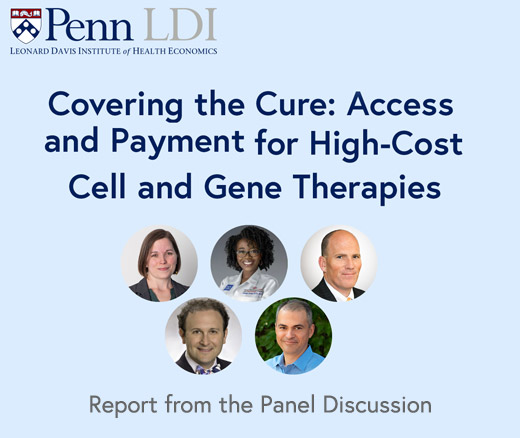
news
health equity
Sustainable funding and equitable access to multi-million dollar gene therapies
Penn LDI panel reveals barriers and options for change
September 19, 2024
blog post
health equity
Medicaid could be a powerful tool to improve racial and ethnic representation in cancer clinical trials
One key may be Medicaid's recent coverage of the routine costs of enrolling in clinical trials.
September 18, 2024
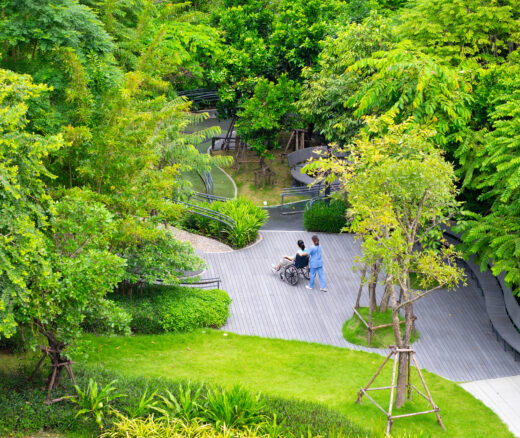
blog post
health equity
The hospital environment itself can be a form of treatment
Patients need a natural room with access to a quiet outdoor environment
September 17, 2024