The initial search retrieved 1944 studies from five databases. After screening and full-text review, 49 studies met the inclusion criteria and were included in the evidence synthesis. Three of these were identified manually from citation searching. Figure 1 shows the identification and screening stages, detailing the reasons for exclusion at full-text screening stage.
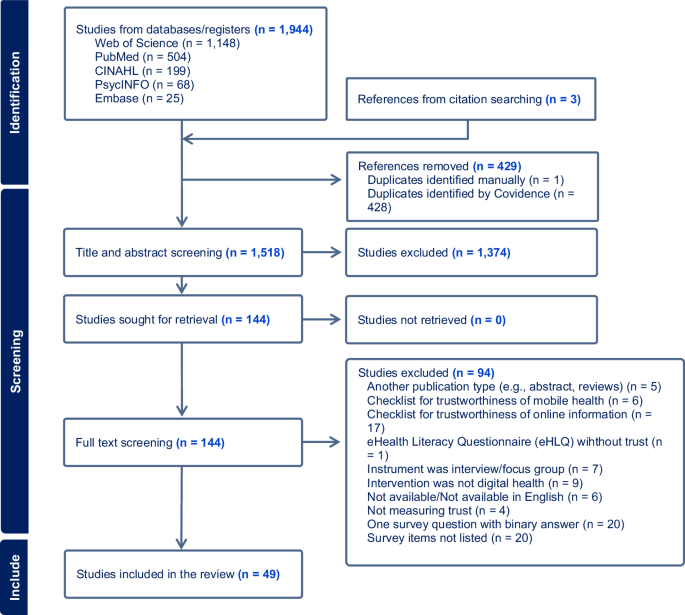
PRISMA diagram including the four steps of identification, screening and final inclusion of studies, with the number of studies included and removed at each stage generated in Covidence.
Study quality
Assessment of the 49 included articles using SQUIRE 2.020 revealed a mean score of 16.6 (out of 18) indicating that studies were high quality overall. Fourteen articles (28.6%) scored 100%, six (12.2%) scored below 80.9%, while the remainder scored between 81.0 and 99.9%. The most common reasons for lower quality scores were lack of description for the approach used to assess the impact of the intervention (n = 18, 36.7%), unclear articulation of ethical aspects (n = 16, 32.6%), absence of a concise summary of key findings (n = 16, 32.6%), and a failure to explicitly disclose study fundings (n = 6, 12.2%).
Studies characteristics
Included studies were published from 2010 to 2023, with approximately a quarter (n = 12, 24.5%) published in 2021. Countries with the highest number of publications were China (n = 12, 24.5%) and the United States (n = 8, 16.3%), followed by Australia, Canada, Italy, Spain, Switzerland and the United Kingdom with two studies (2.1%) each. Study designs comprise cross-sectional survey studies (n = 45, 91.8%), randomised controlled trials (n = 1, 2.5%), field experiments (n = 2, 4.1%), and retrospective observational studies (n = 1, 2.5%). In the cross-sectional studies, 26 (53.1%) used structural equation modelling in their methods.
Participants characteristics and data collection methods
Across all study populations, a total of 26,165 people were surveyed, including patients (n = 20,023; 36 studies), patients and their families (n = 60; 1 study), patients and healthcare professionals (n = 316; 1 study), healthcare professionals (n = 647; 2 studies), digital intervention users (n = 9799; 4 studies) or the general population (n = 2635; 5 studies). Survey data was collected predominantly online (n = 28, 57.1%), on paper (n = 9, 18.4%), or by a combination of data collection methods (n = 7, 14.3%). Five studies (10.2%) did not report the data collection method.
Digital healthcare modalities and interventions
Interventions included various modalities of digital healthcare (chatbots, wearables, sensors, virtual reality, electronic medical records, medical artificial intelligence) (n = 13, 26.5%)21,22,23,24,25,26,27,28,29,30,31,32,33; telehealth (teleconsultation, remote patient monitoring, eConsults) (n = 22, 44.9%)19,34,35,36,37,38,39,40,41,42,43,44,45,46,47,48,49,50,51,52,53,54,55; and mobile health (mobile health apps, mobile management systems, mobile teledermatoscopy) (n = 14, 28.6%)56,57,58,59,60,61,62,63,64,65,66,67,68. A table in Supplementary Information (Supplementary Table 1) details the digital healthcare interventions and presents the terminology adopted by the authors in the included studies.
Definitions of trust
While 21 studies (42.9%) did not define trust, the remaining 28 papers (57.1%) presented a broad variety of definitions. These included several definitions based on trust-related processes measured in the study such as concerns about the accuracy of the data collected (n = 3, 6.1%)29,32,57; the willingness to rely or depend on something or someone (n = 5, 10.2%)40,51,55,65,68; the acceptance of uncertainty and vulnerability based on positive expectations (n = 4, 8.1%)43,49,63,67; the explanation of trust’s role in supporting interpersonal relationships (n = 4, 8.1%)22,30,35,69, data sharing (n = 2, 4.1%)38,46, intention to use (n = 3, 6.1%)37,58,62 or adopt (n = 1, 2%)24 digital healthcare. Other explanations differentiated cognitive trust (developed by observing others performance objectively) (n = 2, 4.1%)28,56, from affective trust (involves emotional and irrational feelings beyond performance observation) (n = 1, 2%)28, initial trust (trust from the first interaction) (n = 2, 4.1%)61,66 and online trust (expectation that ones’ vulnerability will not be attacked online) (n = 1, 2%)43.
Instruments to measure trust in digital healthcare
In 36 studies (73.5%), trust in digital healthcare was measured with a unidimensional instrument, while the remaining instruments (26.5%) had two to five dimensions to measure the construct of trust. Trust in digital healthcare was often measured together with other constructs (e.g., behavioural intention, satisfaction, privacy and security concerns, perceived use and risk, technology anxiety, etc.) (n = 45, 91.8%), and only four studies (8.2%) measured trust in digital healthcare as the unique construct.
Extensions of existing theoretical frameworks such as Technology Acceptance Model (TAM), Unified Theory of Acceptance and Use of Technology (UTAUT and UTAUT2), diffusion of innovation or a combination of those were used in 17 studies (34.7%), while six (12.2%) used the Theory of Reasoned Action (TRA), Theory of Planned Behaviour (TPB), Social Cognitive Theory, the Extended Valence Framework and/or other references to develop their research models. Previously published surveys were adopted in 37 studies (75.5%), including the Patient Trust Assessment Tool (PATAT) questionnaire34,51 and the Trust in Physician Scale19,36. Twelve studies (24.5%) adapted items from non-health-related references, particularly trust measures for e-commerce30,33,45,68,69. Six studies (12.2%) developed their own trust items without mentioning any reference.
Trust domains and items
A table summarising the trust domains and items to measure trust in digital healthcare described in the included studies, identifying those that went through any qualitative and/or quantitative validation processes is presented in Supplementary Information (Supplementary Table 1). Figure 2 presents trust domains identified more than once in the included studies.
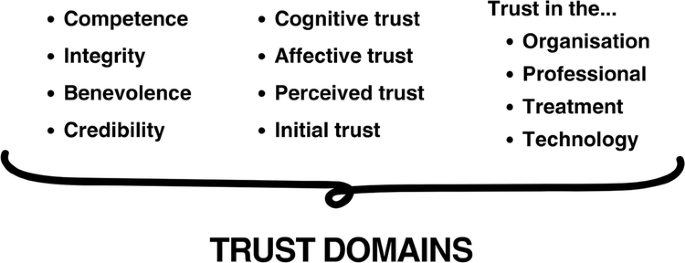
This figure outlines and groups the major domains associated with trust. The first group refers to attributes associated with trust, the second group has different types of trust, while the third includes the entities to which trust is attributed.
Response options
Survey responses predominantly used metrics such as Likert scales with 5-points (n = 30, 61.2%), 7-points (n = 12, 24.5%) and 4-points (n = 3, 6.1%). Other options included binary responses (n = 2, 4.1%) and Likert scales with 6-points (n = 1, 2%) or without stating the number of response categories (n = 1, 2%).
Validation
Twelve (28.5%) studies adopted a qualitative validation stage, using either experts’ feedback or a pilot study with a small sample to evaluate face and content validity, followed by changes to the survey items before data collection. Thirty-two studies (65.3%) conducted quantitative validation, including exploratory and confirmatory factor analysis to evaluate structural validity and other methods to evaluate criterion validity, such as convergent and discriminant validity or Structural Equation Modelling (SEM). In total, eight studies (16.3%) did not report any statistical validation method, claiming the survey used was already validated in other populations or contexts. Four studies (8.2%) did not report any validation method.
Internal consistency
In 29 studies (59.2%), Cronbach’s alpha was used to measure internal consistency reliability of trust scales or subscales, and in 22 studies (44.9%) composite reliability was calculated. Sixteen studies conducted both Cronbach’s alpha and composite reliability (31.6%) and 14 studies (28.6%) did not conduct any internal consistency analysis of trust scales.
Factors and outcome measures associated with trust
Factors associated with trust in digital healthcare included perceived risk43,49,55, privacy concerns with data sharing19,23,38,68, and previous experience54,64. The impact of human presence on trust in digital healthcare was also evaluated in three (6.1%) studies28,31,60. Trust was associated also with digital literacy, education levels, and learning (n = 3, 6.1%)22,39,54. Some studies associated sociodemographic variables with trust, such as income (n = 1, 2%)19, or simply quantified the level of trust in the digital healthcare intervention (n = 4, 8.2%)23,34,39,70.
From the included studies, 20 (40.8%) associated trust with intention to use or to continue to use digital healthcare21,26,32,35,37,40,45,53,62,63,65,67,68,69, five (10.2%) associated trust with adoption, intention to adopt or continued adoption27,43,55,61,66, while two studies (4.1%) associated trust with either acceptance30,33 or usefulness31,49 of digital healthcare interventions as their main outcome.
Factors positively associated with consumers’ trust in digital healthcare
Several factors are associated with consumers’ trust in digital healthcare. The accuracy of data obtained from the digital healthcare intervention has been shown to influence trust29, and trust in health providers, as an information source, optimised consumers’ willingness to share wearable data23. Consumers’ levels of digital literacy and level of education have been identified as predictors of, or have been significantly associated with trust39,54. Similarly, one study found a significant association between previous experience with digital healthcare and trust54, while the same association did not change in a pre and post study64. Consumers’ trust is positively influenced by digital healthcare quality46, while also significantly correlated to satisfaction and learning22. Sociodemographic factors such as consumer income had a significantly positive association with the level of trust in physicians using telemedicine19. Consumers also value personalised or customised digital healthcare services, with both aspects increasing their trust68.
Factors negatively associated with consumers’ trust in digital healthcare
Perceived risk and privacy concerns were found to be negatively associated with trust in digital healthcare43,49,55,68. Mistrust negatively impacts the frequency and willingness to share data online38, whereas digital natives (i.e., people born after 1980) are more worried about data protection, compared to digital immigrants (i.e., people born before 1980)50.
Outcomes positively associated with consumers’ trust in digital healthcare
Over half of the included studies in this review (N = 49) found that consumers’ trust is positively associated with some aspects of digital healthcare. Of the 46 studies measuring trust in digital healthcare from consumers’ perspectives, trust positively affected their intention to use or to continue using digital healthcare in 13 studies (28.2%)32,35,37,40,45,47,48,53,57,58,62,65,69. In three studies (6.5%), trust predicted the intention to use21,63,67, and in one (2.2%) trust did not predict the intention to use digital health49. Another study reported that trust could mediate the paradoxical effect of beneficial and desired personalisation in mobile health versus privacy concerns on the intentions to use this modality of digital healthcare68. Initial trust, online trust, and trust in health providers positively affected consumers’ adoption of digital healthcare43,55,61,66, and in one study, consumers’ trust was a predictor for the adoption of medical AI27. Trust significantly impacted the perceived usefulness of AI-assisted living technology25 and of online fertility consultations and self-collection tests49. Trust was also a strong predictor of electronic medical records acceptance.
Figure 3 summarises on the left-hand side the factors positively and negatively associated to consumers’ trust in digital healthcare and on the right-hand side the outcomes positively associated to consumers’ trust in digital healthcare found in the included studies.
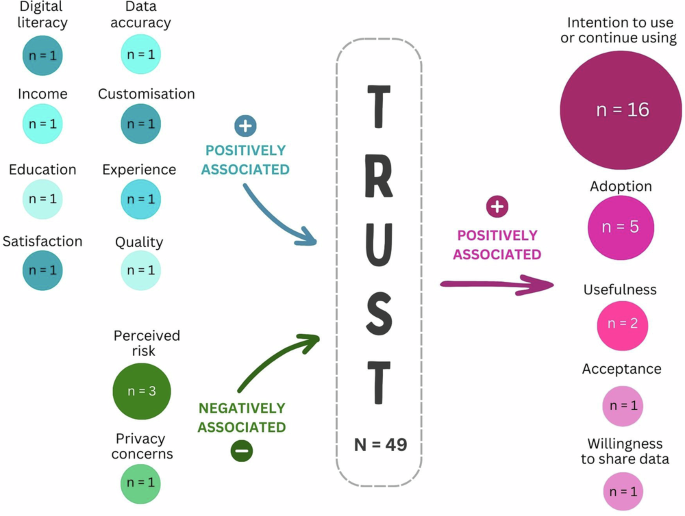
The bubbles on the left-hand side of the figure denote factors associated with trust, while the bubbles on the right-hand side represent outcomes associated with having trust. Each bubble contains the number of identified studies exploring that factor. The arrows explain the direction of the association.
Consumers trust human interactions more than machines
Consumers are less likely to trust AI-goal setting features28, telediagnosis in dermatology60, and virtual reality mindfulness training22, compared to in-person interactions. Consumers also tend to have more trust in AI-enabled digital healthcare if they perceive that there is interaction with a human rather than a virtual agent only31.
Levels of trust in different aspects of digital healthcare
The studies that analysed levels of trust in different aspects of digital healthcare found: consumers tend to trust telemedicine services34, trust providers as a source of health information23,39, and trust a mobile virtual AI-supported virtual agent to perform medical interviews70. Also, trust in the technology, healthcare professionals, and the treatment affects trust in the telemedicine service to manage anticoagulation treatment, whereas trust in the healthcare organisation does not seem to affect this treatment51.
Healthcare professionals’ trust in digital healthcare (n = 3)
The three studies (6.1%) reporting healthcare professionals’ trust in digital healthcare were published in Germany, Spain, and China, and included results from cross-sectional surveys. Altogether, these papers analysed 697 health professionals’ trust in digital healthcare interventions, including mobile health apps56, electronic healthcare records30, and eHealthMonitor (a personalised eHealth platform to accumulate online health data to support decisions on disease treatment or prevention)26. The results indicate that cognitive trust (i.e., developed by observing others performance objectively) can significantly influence the use behaviours of mobile health apps56. Also, provider’s perceptions of the favourable conditions that lead to the success of the digital healthcare resource strongly predict acceptance of electronic record systems30. Educational interventions can improve medical professionals’ trust in data privacy, considered the main hindrance to eHealthMonitor use26.