Healthcare is a data-rich industry ripe for artificial intelligence deployment. The impact of AI in healthcare has been positive.
Hospitals, health systems and other provider-based organizations have integrated AI into their daily workflows to improve patient care, reduce costs and enhance efficiency. Providers, payers and other stakeholders have also realized many other advantages, including more personalized treatment plans, improved communications across stakeholders and digital transformation across the enterprise.
AI is here to stay in healthcare, and its reach will likely increase. Innovations in AI, such as generative AI (GenAI), agentic AI and intelligent automation, are making waves in the healthcare industry, attempting to solve some of healthcare’s most significant pain points.
This list details, in alphabetical order, the top 12 ways AI has and will continue to impact healthcare.
1. Clinical decision support
At its core, a clinical decision support system (CDSS) is a critical tool designed to improve care quality and patient safety. But technologies such as AI and machine learning are transforming clinical decision-making.
In the early days of CDSS tools, many were standalone offerings that were not well integrated into clinical workflows. Today, many CDSSes are integrated into electronic health records (EHRs) to help improve deployment and gain more value from the use of these tools at the bedside.
AI takes this one step further by enabling providers to take advantage of information within the EHR and data pulled from outside of it. Because AI tools can process larger amounts of data more efficiently than other tools while enabling stakeholders to pull fine-grained insights, they have significant potential to transform clinical decision-making.
Using AI’s advanced pattern recognition capabilities, CDSS tools can incorporate risk stratification and predictive analytics to help clinicians make more informed, personalized treatment recommendations in high-value use cases, such as chronic disease management.
2. Drug discovery and development
Drug discovery, development and manufacturing have created new treatment options for various health conditions. Integrating AI and other technologies into these processes will continue revolutionizing the pharmaceutical industry.
High drug development costs and other challenges are driving clinical researchers to seek out new tools to get new drugs to market more efficiently. The process is often high risk, high reward: The drug development lifecycle takes billions of dollars and decades of research, but new medicines aren’t guaranteed to receive regulatory approval from the U.S. Food and Drug Administration.
AI and other technologies can help overcome major drug discovery and development barriers.
AI and machine learning, in particular, are revolutionizing drug manufacturing by enhancing process optimization, predictive maintenance and quality control while flagging data patterns a human might miss, improving efficiency.
These tools are also helpful in the data-gathering systems for complex drug manufacturing, and models to identify novel drug targets are reducing the time and resource investment required for drug discovery.
According to pharmaceutical developer Roche, AI is instrumental in creating a more feasible and sustainable timeline for drug development.
“The sheer scale and complexity of the scientific data involved in drug discovery pose significant barriers to progress,” the company wrote in a Jan. 30, 2025, post. “Computational approaches have enhanced data collection and analysis, but have historically not matched the magnitude of this problem. Thus, there’s still potential for further advancements in the faster delivery of new medicines and improved success rates in research.”
Investments in AI are paying off for pharmaceutical companies. A study published in the June 2024 issue of Drug Discovery Today revealed that AI discovered molecules far better than historic industry averages. The study’s authors suggested that scientists should continue to measure their success as AI-discovered molecules continue down the development pipeline.
3. Electronic health records
EHRs hold vast information about a patient’s health and well-being in structured and unstructured formats. This data is valuable for clinicians — but making it accessible and actionable has challenged health systems.
AI has given healthcare organizations a unique opportunity to overcome some of these hurdles, and some already see the benefits.
EHR adoption aims to streamline clinical workflows while bolstering cost-effective care delivery. However, clinicians cite clinical documentation and administrative tasks as EHR burdens and sources of burnout.
AI tools are key to addressing these issues and giving providers back their time so they can focus on patients. There are multiple AI use cases to tackle clinician burnout, most of which aim to automate aspects of the EHR workflow.
Health data extraction products can help clinicians find the information they’re looking for quickly and effectively, reducing information overload. Many of these tools use natural language processing (NLP). This AI approach enables algorithms to flag key components of human language and use those insights to parse through text data to extract meaning.
AI is also beneficial when healthcare organizations move to new EHR platforms and must undertake legacy data conversion. This process often reveals that patient records are missing, incomplete or inconsistent, which can create significant inefficiencies.
Typically, inconsistencies pulled from a medical record require data translation to convert the information into the language of the EHR. The process usually requires humans to translate the data manually, which is time-consuming and labor-intensive and can also introduce new errors that could threaten patient safety.
AI-based tools can automate this process, saving time and effort for care teams.
Finally, ambient documentation systems powered by AI are instrumental in streamlining provider documentation burdens. Using NLP and machine learning, these tools “listen” to patient-provider conversations during the clinical encounter, transcribe them and then generate a clinical note filed into the EHR for provider review.
4. Genomics
Genomics has sparked a wealth of excitement across the healthcare and life sciences industries. Genetic data lets researchers and clinicians better understand what drives patient outcomes, potentially improving care.
Particularly, genomics plays a key role in precision and personalized medicine, but making these insights useful requires analyzing large, complex data sets.
By enabling providers to combine the power of genomics and big data analytics, AI models can tailor care and treatment recommendations for various medical conditions. These tools are invaluable for overcoming a significant obstacle to using genomics in clinical settings: the data’s actionability.
Access to a patient’s genome sequence data sounds promising, as genetic information is relevant to identifying potential health concerns, such as hereditary disease. However, to truly transform care delivery, providers need to know more than just what the data says about a patient’s genetic makeup. They must also determine how that information can be used in the real world.
One approach to achieving this involves integrating genomic data into EHRs, which can help providers access and evaluate a more complete picture of a patient’s health. But AI can take this further.
“Artificial Intelligence (AI) is valuable in genomics because it enables researchers to analyse vast amounts of complex genomic data more efficiently and accurately than before,” according to an Oct. 17, 2024, blog post by Katrina Costa, a science writer at the Wellcome Sanger Institute. “For example, each human genome contains around 3 billion base pairs and large-scale studies can involve hundreds of thousands of genomes. AI can also help identify patterns and correlations in data that are too subtle or complex for us to detect, and predict the impact of specific changes.”
A study published in the May 20, 2024, issue of Nature Communications detailed how an AI-driven model used genomics and epigenetics to assess risk for certain autoimmune diseases. To flag genetic mutations causing certain illnesses, medical researchers must distinguish between cell types — something that’s not always possible. Using an AI-powered tool and genomics, the researchers could predict disease more accurately and thus intervene sooner.
5. Hospital management
Managing health system operations is at the heart of how healthcare is delivered. Optimizing workflows and monitoring capacity can have major implications for a healthcare organization’s bottom line and its ability to provide high-quality care.
However, monitoring and managing all the resources required is no small undertaking, and health systems are increasingly looking to data analytics tools such as AI to help.
Capacity management is a significant challenge for health systems, as issues like ongoing staffing shortages and recent surges in respiratory viruses can exacerbate existing hospital management challenges. Many hospitals, such as Cleveland Clinic, have implemented smart scheduling that uses AI to analyze historical data — including patient volume trends and staff availability — to optimize shift rosters. This type of scheduling can also predict when more staff might be needed, such as during peak flu season and holidays.
AI-enabled capacity management is beneficial for surgical scheduling. Since operating rooms are high-cost, high-demand hospital areas, AI can minimize OR downtime by optimizing procedure scheduling and staff availability.
Some hospitals have also started using digital twins to improve operational management and performance.
Digital twins are virtual replicas of a hospital, including its typical patients, workflows and departments. The technology mirrors data from the EHR, real-time solutions and other IT systems to provide hospital leaders with a platform to test changes and how they might affect care delivery. According to a March 22, 2024, article in npj Digital Medicine, typical applications in healthcare include hospital management, facility design, workflow development, decision-making and individualized therapy.
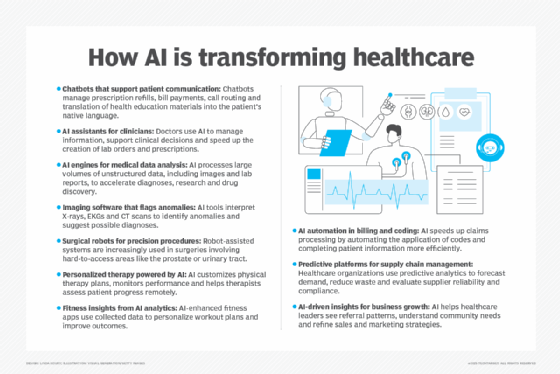
6. Medical imaging
Medical imaging is critical in diagnostics and pathology, but effectively interpreting these images requires significant clinical expertise and experience. Imaging analytics, often driven by AI, aims to tackle this.
AI technologies are already changing medical imaging by enhancing screening, risk assessment and precision medicine.
In a study published in the March 11, 2024, issue of Communications Medicine, Johns Hopkins researchers showed that a deep neural network-based automated detection tool could assist emergency room clinicians in diagnosing COVID-19 by analyzing lung ultrasound images.
The tool is designed to identify B-lines — bright, vertical image abnormalities that indicate inflammation in patients with pulmonary complications — with a high degree of accuracy to diagnose COVID-19 infection.
The model’s success suggests that a similar approach could be applied to other severe conditions, such as heart failure, to diagnose patients efficiently at the point of care.
The researchers emphasized that such a capability would be instrumental in scenarios where emergency department clinicians face high caseloads, like during flu and COVID seasons, or for integration into wearable technologies and other wireless devices for enhanced remote patient monitoring.
AI can improve every aspect of a radiologist’s workflow, a top priority for healthcare organizations as the demand for radiologists is expected to grow by almost 26% between 2023 and 2055, according to a study from the Harvey L. Neiman Health Policy Institute published in the February 2025 issue of the Journal of the American College of Radiology.
7. Medical research and clinical trials
Medical research is a cornerstone of the healthcare industry, facilitating the development of game-changing treatments and therapies. But this research, particularly clinical trials, requires vast amounts of money, time and resources.
AI tools can help researchers overcome the top challenges of clinical trials, including the time it takes to recruit or match patients to a trial, collect large amounts of data from various sources and manually analyze data.
AI-powered chatbots can be especially useful for clinical trials to guide patients through eligibility screening and onboarding. These technologies are particularly valuable for accelerating clinical trials by improving trial design, optimizing eligibility screening and enhancing recruitment workflows.
Further, AI models can help advance clinical trial data analysis, as they enable researchers to process extensive data sets, detect patterns, predict results and propose treatment strategies informed by patient data. AI has also proven helpful for trial design, enabling protocol simulation to reduce costly amendments.
8. Patient engagement
Patient engagement significantly improves health outcomes by enabling patients and their loved ones to be actively involved in care. Patient engagement solutions are often designed to balance convenience and high-quality interpersonal interaction.
While digital technologies cannot replace the human elements of the patient experience, they have their place in healthcare consumerism. AI, specifically, can be valuable for personalizing patient engagement tools.
Communication is a key aspect of patient experience and activation. EHRs can help facilitate that communication by allowing patients and providers to send messages to one another using the patient portal. However, overflowing inboxes can contribute to clinician burnout, and some queries can be too complex or time-consuming to address using an EHR message.
This creates frustration on both sides, as clinicians want to spend more time on care and less on administrative tasks, while patients want their healthcare to be accessible and frictionless.
AI chatbots are emerging as a potential solution to this conundrum. They are well-suited to analyzing patient needs and providing resources in certain areas.
For example, GenAI is being embedded into more patient portals to address the following two tasks:
Mining patient messages and triaging them to the appropriate clinical team member.
Fielding patient messages, analyzing them and generating a response.
Studies have shown that GenAI tools provide good medical advice in patient portal messages, although their responses still require review by a healthcare provider.
What’s more, chatbots can help filter patient phone calls, sifting out those that can be resolved by providing basic information, such as giving parking information to hospital visitors. The emergence of agentic AI takes this a step further by helping to complete these administrative tasks. These AI tools can also be applied to clinical needs, using patient symptom data to provide care recommendations.
AI-driven patient engagement can also take the form of tools designed to conduct patient outreach based on clinical risk assessment data or systems to translate health information for users in a patient portal.
9. Predictive analytics and risk stratification
In recent years, the rise of predictive analytics has aided providers in delivering more proactive healthcare to patients. In the era of value-based care, the capability to forecast outcomes is invaluable for developing crucial interventions and guiding clinical decision-making.
To successfully use predictive analytics, stakeholders must be able to process vast amounts of high-quality data from multiple sources. For this reason, many predictive modeling tools incorporate AI in some way, and AI-driven predictive analytics technologies have various benefits and high-value use cases.
Predictive analytics enables improved clinical decision support, population health management and value-based care delivery, and its healthcare applications are continually expanding.
AI-based risk stratification is a crucial component of many of these efforts, as flagging patients at risk for adverse outcomes and preventing those outcomes is integral to advancing high-quality care delivery.
For example, researchers at the University Medical Center Groningen developed an AI-driven model to stratify the risk of coronary artery disease (CAD). The model uses an AI-powered questionnaire to forecast a person’s 10-year CAD risk by analyzing their answers about lifestyle, medical history and social factors.
The model proved to be as accurate as traditional clinical risk tools that require laboratory analyses, reducing unnecessary utilization. Researchers also touted the cost-effectiveness and scalability of the AI-powered questionnaire, which is more accessible to patients than lab tests.
Hospitals are also applying AI capabilities to established predictive analytics solutions that predict adverse events before they happen. Top use cases in this area include risk assessment for sepsis, heart failure and hospital readmissions.
10. Remote patient monitoring
Remote patient monitoring (RPM) has become more familiar to patients following the COVID-19 pandemic and the resulting rise in telehealth and virtual care. However, RPM technologies present significant opportunities to enhance patient well-being and improve care by enabling providers and researchers to use additional patient-generated health data.
AI can be incorporated into RPM tools or used to streamline RPM data processing.
Common RPM tools that use advanced analytics approaches like AI play a significant role in advancing hospital-at-home programs. These initiatives let patients receive care outside the hospital setting, necessitating that clinical decision-making must rely on real-time patient data.
RPM offerings enable continuous and intermittent recording and transmission of this data. Tools such as biosensors and wearables are frequently used to help care teams gain insights into a patient’s vital signs or activity levels.
AI bolsters these tools’ capabilities by helping to predict complications, helping care teams to preemptively intervene in cases of clinical deterioration, and flagging patients who are likely to benefit from hospital-at-home services compared to inpatient care.
These technologies are also helpful because they can learn a patient’s baseline biometrics, detect deviations from that baseline and adjust accordingly or alert the care team when a patient is at high risk for an adverse event.
11. Revenue cycle management
Revenue cycle management (RCM) ensures that health systems can focus on providing high-quality patient care. However, effectively tackling revenue challenges and optimizing operations requires heavy lifting on the administrative side.
AI tools can help ease these burdens in a variety of ways.
RCM still relies heavily on manual processes, but recent trends in AI adoption show that stakeholders are looking at the potential of advanced technologies for automation.
Providers are investigating AI-based tools to streamline claims management, which is rife with labor- and resource-intensive tasks, such as managing denials and medical coding. To that end, many in healthcare are interested in AI-enabled autonomous coding, patient estimate automation and prior authorization technology.
Healthcare organizations are seeking more information on their ROI before adopting these tools. However, adoption will likely center on operational optimization, leading to automation tools deployed in areas with the highest administrative burden, such as claims management.
AI technologies can take over mundane, repetitive tasks — such as checking a claim’s status — and enable staff to focus on more complex revenue cycle management objectives.
Revenue cycle management has also been a top target for GenAI in healthcare, considering the relatively low risks of applying the newer technology to administrative versus clinical tasks. For example, GenAI can be used for appointment reminders, preauthorization updates, payment reminders and insurance claim updates.
GenAI has also been tapped to improve medical coding by validating codes based on clinical documentation and EHR data and using natural language to turn unstructured data into structured, billing-ready information. Similarly, providers are starting to use GenAI to draft appeal letters for claim denials management, with some AI tools now able to customize denial workflows by payer.
12. Robotics
In healthcare, having another pair of hands when completing various care-related tasks is often helpful. However, with ongoing healthcare workforce shortages, having enough staff to do the critical work of patient care is challenging.
The rise of advanced tools such as AI has shown promise in addressing some of the challenges associated with staffing shortages. Still, concerns about bias, health equity and clinician overreliance on these tools highlight the importance of the human aspect of healthcare.
AI and other healthcare offerings can’t replace humans, but as these tools continue to advance, they’re showing increasing promise in augmenting the performance of the healthcare workforce.
Robotics has generated significant attention recently. To date, many of the applications for healthcare robots are surgical. For example, surgeons can use robotic arms to conduct procedures, allowing for improved dexterity and range of motion.
AI can enhance robotics in surgery, such as the da Vinci Surgical System, which can learn from procedures to improve accuracy and assist surgeons with real-time decision support. Research shows surgeons have primarily used AI for intraoperative enhancements, including optimized force, detection of positive surgical margins and complete automation of specific steps.
Healthcare robots with AI capabilities are also helping patients and providers. Common examples of AI-assisted robots include those such as Diligent Robotics’ Moxi, which helps clinical staff with non-patient-facing tasks, such as running patient supplies, delivering lab samples and fetching items. Patient-facing robots also exist to interact with patients, assist with patient rehabilitation and alert providers to changes in patient status.
These examples are just the beginning of how AI is poised to transform the healthcare industry, and many more changes are likely to emerge as these technologies advance to improve care delivery and patient outcomes.
Editor’s note: This article was updated in May 2025 to include more recent research and to improve the reader experience.
Sara Heath has been covering news related to patient engagement and health equity since 2015.
Jacqueline LaPointe is a graduate of Brandeis University and King’s College London. She has been writing about healthcare finance and revenue cycle management since 2016.